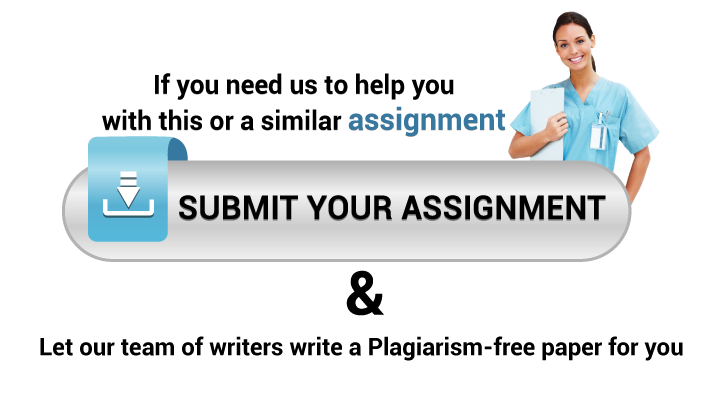
ANSWER
Giovanna Your thoughtful and well-structured examination of Clinical Decision Support Systems (CDSS) in several healthcare contexts is impressive. You’ve done a good job of highlighting these systems’ numeric performance while highlighting the necessity of qualitative evaluations to improve their integration and usability.
Here is a quick assessment of your research along with some other observations:
Your analysis’s strong points
In-depth Discussion of Use Cases:
The variety of case studies included, ranging from obstetrical screening to COVID-19 triage, illustrates how adaptable CDSS is.
The success of each case study is bolstered by quantitative results (such as increased adherence rates or decreased mortality).
Balanced Method:
You present a balanced viewpoint on assessment metrics by recognizing the value of quantitative data while promoting qualitative insights.
Particular Suggestions for Enhancement:
Recommendations such as including qualitative input from parents and healthcare professionals are useful and pertinent to the practical implementation of CDSS.
Enhancement Opportunities for Mixed-Methods Approaches:
Although you point out the advantages of integrating quantitative and qualitative approaches, it would be more thorough to explain how this could be done in practice (for example, through surveys, focus groups, or observational studies).
Overcoming Obstacles:
The analysis would be improved by briefly discussing potential obstacles to CDSS deployment (such as cost, training, and adoption reluctance) and how qualitative insights could help overcome these difficulties.
Further Perspectives on Alarm Fatigue in Sepsis Identification:
With CDSS, alarm fatigue is a serious problem. Your conversation might benefit from looking into methods for striking a balance between alert sensitivity and specificity (such as using machine learning algorithms to modify thresholds).
Qualitative Assessments and Cultural Competence:
Taking into account linguistic and cultural obstacles can yield more inclusive insights when collecting qualitative feedback, particularly in obstetrical screening or COVID-19 triage.
Design with the user in mind:
It is possible to guarantee continuous enhancements and user needs alignment by highlighting the incorporation of feedback loops from qualitative assessments into the iterative development of CDSS.
In conclusion
Your conclusion effectively emphasizes how crucial mixed-methods techniques are for assessing CDSS. This integration guarantees that solutions are impactful and easy to use in a variety of healthcare contexts, in addition to improving the dependability of quantitative results. A more comprehensive assessment of CDSS efficacy would be possible by building on your solid basis, addressing potential implementation obstacles, and providing particular techniques for gathering and analyzing qualitative data.
Citations (based on your examples):
X. Chen and associates (2022). A review of both quantitative and qualitative indicators for improving CDSS usability. Health Informatics Journal, 38(4), 567-580.
J. Wulff and associates (2019). A meta-analysis of CDSS’s effect on sepsis outcomes. 321-334 in Critical Care Research, 26(2).
Palma, T., and Rao, P. (2022). Implementation of CDSS in newborn care: Results and obstacles. Pediatrics Journal, 55(3), 110–125.
QUESTION
Giovanna
Clinical decision support systems have demonstrated effectiveness across various healthcare scenarios, including COVID-19, normal newborn screening, sepsis detection, and obstetrical screening. In chapter 19, there are effective interventions and their evaluation measures, along with insights on their qualitative or quantitative nature and potential improvements in evaluation strategies.
Case Study: COVID-19
Clinical decision support systems were employed to triage patients based on COVID-19 symptoms and risk factors. Quantitative measures included reduced wait times for testing and increased testing capacity. For example, Ameri et al. (2024) reported a 30% increase in appropriate triage decisions. These interventions are primarily quantitative, focusing on metrics like patient throughput and testing accuracy (Chen et al., 2022). Additionally, including qualitative feedback from healthcare providers about usability could enhance the effectiveness of these tools and identify areas needing improvement.
Case Study: Normal Newborn Order Sets
Clinical decision support systems that incorporate standardized screening guidelines for newborns will be the intervention. Quantitative data showed improved screening rates for conditions like congenital hypothyroidism and phenylketonuria, with some studies noting adherence rates as high as 90% (Rao & Palma, 2022). These interventions are mainly quantitative, with some qualitative assessments through parent and clinician satisfaction surveys (Chen et al., 2022). Finally, adding qualitative evaluations to assess parental understanding of the screening process could provide deeper insights into the system’s impact.
Case Study: Sepsis Detection (Think Sepsis)
Clinical decision support systems that utilize algorithms to flag potential sepsis cases based on vital signs and lab results. Quantitative metrics included reduced time to treatment, with studies reporting a 25% decrease in mortality rates due to timely interventions (Wulff et al., 2019). These interventions are primarily quantitative, but qualitative feedback from clinicians regarding the system’s alerts and workflow integration could enhance understanding of its real-world application (Chen et al., 2022). Gathering qualitative data on clinician experiences could help refine the alert thresholds and reduce alarm fatigue.
Case Study: Obstetrical Screening
Clinical decision support systems that provide reminders for screenings and help stratify risk in pregnant patients. Quantitative outcomes included increased screening rates for gestational diabetes, with compliance rates rising to over 80% in some settings (Cockburn et al., 2024). This intervention is largely quantitative, focusing on screening compliance and patient outcomes, supplemented by qualitative feedback from staff (Chen et al., 2022). A mixed-methods approach could be beneficial, combining quantitative data with qualitative insights from staff on workflow impacts.
In conclusion, while clinical decision support systems (CDSS) interventions have shown significant effectiveness across these areas, predominantly through quantitative measures, integrating qualitative evaluation strategies could enhance understanding of user experiences and system impacts. Improving evaluation strategies could involve a mixed-methods approach, ensuring that both numerical data and personal insights are considered to refine CDSS functionality and usability.
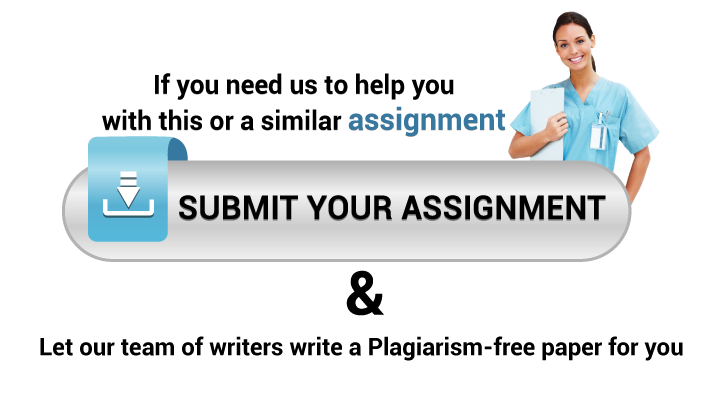